Put Data Scientists in a Position to Succeed
Asking the right questions in analytics. Secured credit cards and financial inclusion.
Asking the Wrong Questions
In 2012, Thomas Davenport and D.J. Patil wrote an article for Harvard Business Review titled Data Scientist: The Sexiest Job of the 21st Century, in which they introduced a new type of “professional with the training and curiosity to make discoveries in the world of big data”.
Thus, the new hottest job title was born: Data Scientist.
(it helped immensely that D.J. Patil was the Head of Data Products and Chief Scientist at LinkedIn, where new and sometimes nonsensical job titles are born daily)
The dominant trait possessed by data scientists, according to Davenport and Patil, is an intense curiosity:
a desire to go beneath the surface of a problem, find the questions at its heart, and distill them into a very clear set of hypotheses that can be tested.
Which is why this new title fits so well:
Perhaps it’s becoming clear why the word “scientist” fits this emerging role. Experimental physicists, for example, also have to design equipment, gather data, conduct multiple experiments, and communicate their results. Thus, companies looking for people who can work with complex data have had good luck recruiting among those with educational and work backgrounds in the physical or social sciences. Some of the best and brightest data scientists are PhDs in esoteric fields like ecology and systems biology.
And this has come to define the modern conception of the role—a curious, unbiased individual sitting in the big data equivalent of a lab; where hypotheses are created, tested via controlled experimentation, and proven or disproven.
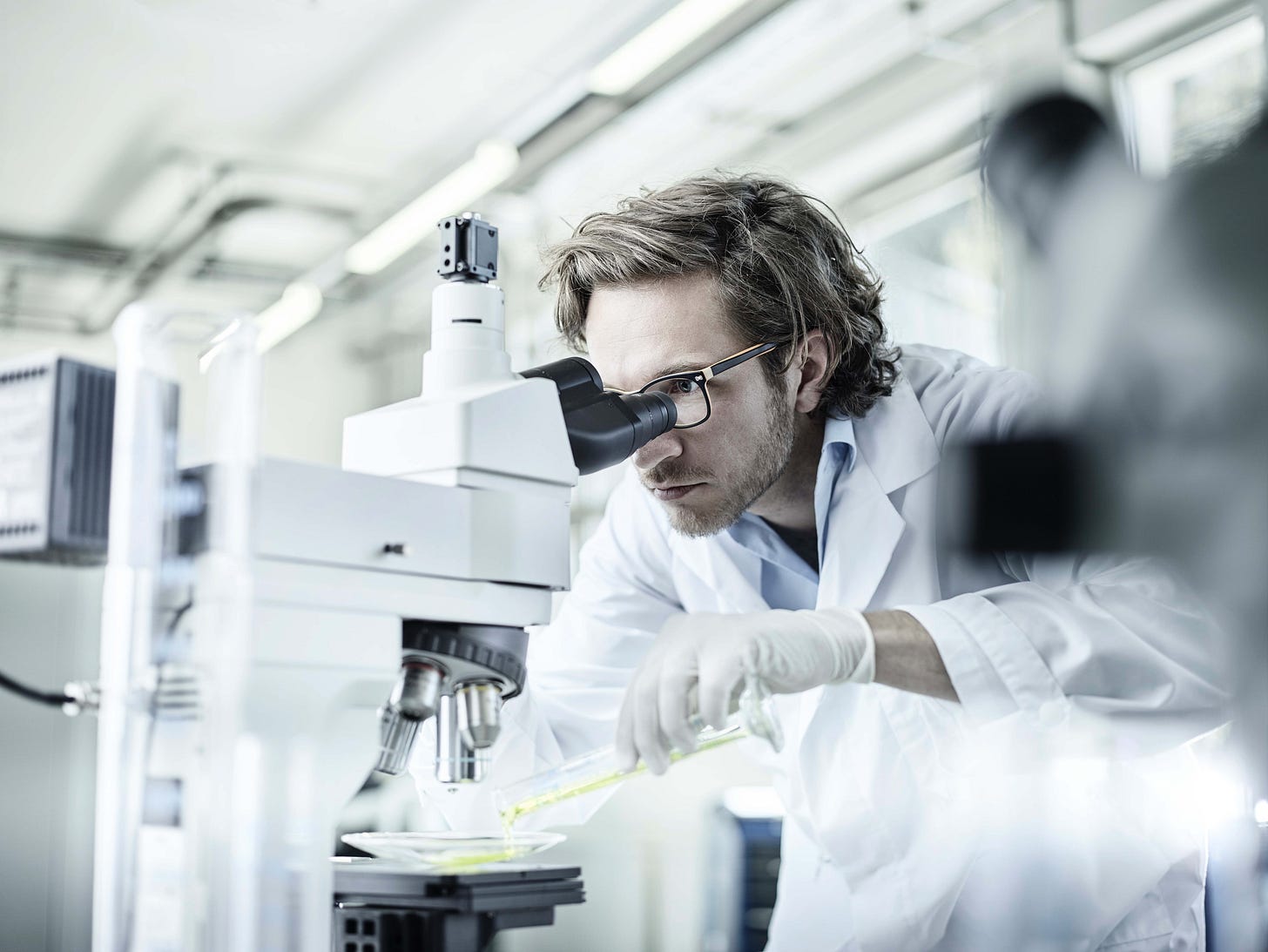
In an academic setting, scientists rely on their own observations about the world to guide their research. They see something that doesn’t make sense. They develop a new hypothesis to help them figure it out. And they attempt to prove or disprove that hypothesis by running experiments and measuring the results.
In a business setting, data scientists don’t have this same luxury. They work for a company that is trying to create value for customers and shareholders. They apply the same scientific process to discovering answers to questions, but the questions themselves are assigned to them by business executives.
And this is where organizations can get into trouble, because if they ask data scientists the wrong questions, they will end up making wrong decisions.
I’ll let Jonah Hill explain:
In the financial services industry, we’ve asked data scientists some great questions, which have produced some outstanding predictive models and decision strategies.
We’ve also had a few misses.
A good example (which has been on my mind lately) is the subtle, yet important distinction between negative credit files and thin/missing credit files.
If you give a data scientist access to consumer credit history data and ask them to predict which consumers will make on time payments on a loan, they can build you a scoring model that will very accurately sort goods from bads. In trying to help you answer the question, the data scientist might also suggest a specific threshold under which an applicant would be considered too high risk to be approved for a loan.
In this model, an applicant with a negative credit history (and thus a low score) would receive the same decision as an applicant with little or no credit history (and thus no score).
But of course, an applicant with a low credit score and an applicant without enough data to calculate a credit score are two entirely different things. The former is a demonstrably high risk. The latter is an unknown — a student, a recent immigrant, a habitual user of cash and debit cards — and unknowns can and should be seen as opportunities in the right environment.
To more effectively address the opportunity that these unknowns present, we need to start by asking the right question — how can we build profitable relationships with consumers who are new to credit?
Fortunately, there are a few financial services providers who have started asking that exact question:
Amazon’s Credit Builder Card, issued by Synchrony, is a secured credit card that offers a surprisingly generous 5% cash back on all Amazon purchases for Prime members (the same reward rate as Amazon’s other credit cards). The card requires a security deposit of $100 to $1,000 (based on risk), but allows customers to upgrade to a regular Amazon credit card in as quickly as seven months (assuming good payment history).
Petal, a fintech start-up that recently raised a $300 million debt round, has partnered with WebBank to issue credit cards nationwide to consumers who Petal’s CEO calls “invisible prime”. The company has roughly 50,000 customers today, a majority of whom are millennials or Gen Z.
Navy Federal Credit Union has offered a secured credit card for a while, but the company recently took a renewed interest in the product based on rising competition from fintechs.
Navy Federal increased its marketing outreach to this audience, but didn't see any increase in secured card acquisition, [Justin] Zeidman [head of credit card products at Navy Federal Credit Union] said...Then it realized that its minimum deposit requirement of $500 was too high for those members.
"They didn't have a lot of deposits on the books with us," Zeidman said. "On average we saw $700 … you're asking them to shove over 75% of their savings, just so they can establish credit."
To make its card more appealing, Navy Federal lowered the secured credit line to a $200 deposit — and saw acquisitions nearly double.
Its next step was to look at graduation. Most of the secured cardholders, upon graduation, were applying for a different Navy Federal card. The credit union chose to instead upgrade those members to its standard cash-back card.
"I know it's no secret secured credit isn't the most profitable place to play, but when you think about the lifetime value of being the first issuer to lend to someone — and then the value of that loyalty over the course of years and years — for us it made a lot of sense," Zeidman said.
That last point is key. There is a long-term benefit to building an environment that nurtures the growth of potentially profitable customers. Analytics can play a pivotal role in such an environment, but it requires us to ask the right questions.
Additional Reading:
The term “Data Science” goes back quite a ways. Here’s a short history.
FICO’s Tim VanTassel paraphrased one of my all-time favorite movie quotes to illustrate the perils of investing in analytics without having a plan in place to operationalize those analytics:
“Your data scientists are so preoccupied with whether or not they can predict something, they don’t stop to consider what will happen if they are successful.”
Petal’s belief in the existence of the “invisible prime” is supported by the data. According to Larry Santucci, senior research fellow for the consumer finance institute at the Federal Reserve Bank of Philadelphia, roughly 25% of secured credit card customers turn out to be super-prime once they have demonstrated sufficient credit performance.
Between the integration of alternative data — in the U.S. and abroad — and the incorporation of consumer-permissioned deposit data, FICO is asking a lot of the right questions about financial inclusion.
One of my all-time favorite analyst reports — The Six Million Dollar Customer: Using Technology to Build a Profitable Customer Base — written by Ben Jackson (@BensAnalysis) during his time at Mercator Advisory Group (@MercatorAdvisor) provides a very comprehensive overview of how financial service providers can use technology and analytics to shape the development of customers’ behavior and profitability.
Did you know?
Neil Armstrong applied for a Diners Club credit card and was rejected almost exactly five years after he walked on the moon?
Talk about your “invisible primes”.
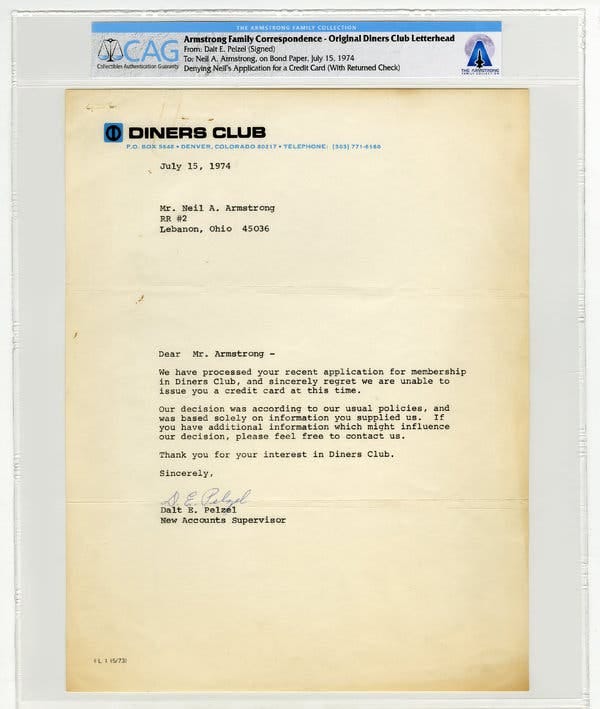
Thanks,
Alex Johnson